Contents
- TF-Agents 모듈 임포트하기
- TF-Agents 환경 준비하기
- TF-Agents 환경 살펴보기
- TF-Agents 행동 반영하기
- TF-Agents 환경 Wrapper 사용하기
- TF-Agents 에이전트 구성하기
- TF-Agents 정책 다루기
- TF-Agents 정책 평가하기
- TF-Agents 데이터 수집하기
- TF-Agents 에이전트 훈련하기
Tutorials
- Python Tutorial
- NumPy Tutorial
- Matplotlib Tutorial
- PyQt5 Tutorial
- BeautifulSoup Tutorial
- xlrd/xlwt Tutorial
- Pillow Tutorial
- Googletrans Tutorial
- PyWin32 Tutorial
- PyAutoGUI Tutorial
- Pyperclip Tutorial
- TensorFlow Tutorial
- Tips and Examples
TF-Agents 데이터 수집하기¶
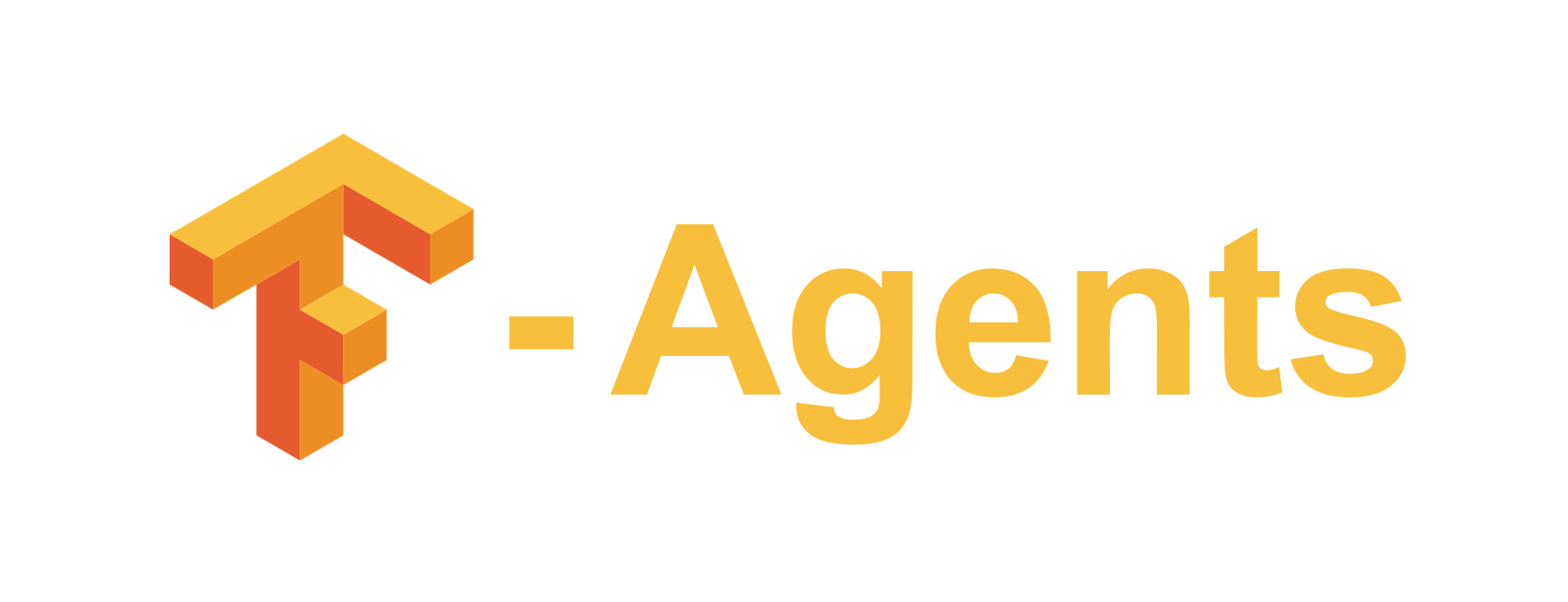
Replay Buffer는 강화학습의 환경으로부터 수집한 데이터를 활용하기 위해 사용합니다.
이번에는 Replay Buffer를 만들고, 주어진 환경과 정책으로부터 데이터를 수집하는 과정에 대해 소개합니다.
■ Table of Contents
1) Replay Buffer 만들기¶
예제1¶
import tensorflow as tf
from tf_agents.environments import suite_gym
from tf_agents.environments import tf_py_environment
from tf_agents.agents.dqn import dqn_agent
from tf_agents.networks import q_network
from tf_agents.utils import common
from tf_agents.policies import random_tf_policy
from tf_agents.replay_buffers import tf_uniform_replay_buffer
from tf_agents.trajectories import trajectory
env_name = 'CartPole-v0'
train_py_env = suite_gym.load(env_name)
eval_py_env = suite_gym.load(env_name)
train_env = tf_py_environment.TFPyEnvironment(train_py_env)
eval_env = tf_py_environment.TFPyEnvironment(eval_py_env)
q_net = q_network.QNetwork(
train_env.observation_spec(),
train_env.action_spec(),
fc_layer_params=(100,))
optimizer = tf.compat.v1.train.AdamOptimizer(learning_rate=1e-3)
train_step_counter = tf.Variable(0)
agent = dqn_agent.DqnAgent(
train_env.time_step_spec(),
train_env.action_spec(),
q_network=q_net,
optimizer=optimizer,
td_errors_loss_fn=common.element_wise_squared_loss,
train_step_counter=train_step_counter)
agent.initialize()
eval_policy = agent.policy
collect_policy = agent.collect_policy
random_policy = random_tf_policy.RandomTFPolicy(train_env.time_step_spec(),
train_env.action_spec())
def compute_avg_return(environment, policy, num_episodes=10):
total_return = 0.0
for _ in range(num_episodes):
time_step = environment.reset()
episode_return = 0.0
while not time_step.is_last():
action_step = policy.action(time_step)
time_step = environment.step(action_step.action)
episode_return += time_step.reward
total_return += episode_return
avg_return = total_return / num_episodes
return avg_return.numpy()[0]
replay_buffer_max_length = 100000
replay_buffer = tf_uniform_replay_buffer.TFUniformReplayBuffer(
data_spec=agent.collect_data_spec,
batch_size=train_env.batch_size,
max_length=replay_buffer_max_length)
tf_agents.replay_buffer.tf_uniform_replay_buffer 모듈의 TFPyEnvironment 클래스는 가장 일반적인 Replay Buffer이며,
배치 단위의 데이터를 수집하고, 균일한 데이터 샘플링을 구현합니다.
예제2¶
print(type(agent.collect_data_spec))
print(agent.collect_data_spec)
print(agent.collect_data_spec._fields)
<class 'tf_agents.trajectories.trajectory.Trajectory'>
Trajectory(step_type=TensorSpec(shape=(), dtype=tf.int32, name='step_type'), observation=BoundedTensorSpec(shape=(4,), dtype=tf.float32, name='observation', minimum=array([-4.8000002e+00, -3.4028235e+38, -4.1887903e-01, -3.4028235e+38],
dtype=float32), maximum=array([4.8000002e+00, 3.4028235e+38, 4.1887903e-01, 3.4028235e+38],
dtype=float32)), action=BoundedTensorSpec(shape=(), dtype=tf.int64, name='action', minimum=array(0), maximum=array(1)), policy_info=(), next_step_type=TensorSpec(shape=(), dtype=tf.int32, name='step_type'), reward=TensorSpec(shape=(), dtype=tf.float32, name='reward'), discount=BoundedTensorSpec(shape=(), dtype=tf.float32, name='discount', minimum=array(0., dtype=float32), maximum=array(1., dtype=float32)))
('step_type', 'observation', 'action', 'policy_info', 'next_step_type', 'reward', 'discount')
data_spec 인자는 Replay Buffer가 수집하는 데이터의 사양을 지정합니다.
agent.collect_data_spec은 에이전트의 관찰 (Observation), 행동 (Action), 보상 (Reward)에 관한 사양을 포함하는 Trajectory 객체입니다.
agent.collect_data_spec._fields를 사용해서 포함된 항목들을 확인할 수 있습니다.
2) 데이터 수집하기¶
예제¶
def collect_step(environment, policy, buffer):
time_step = environment.current_time_step()
action_step = policy.action(time_step)
next_time_step = environment.step(action_step.action)
traj = trajectory.from_transition(time_step, action_step, next_time_step)
buffer.add_batch(traj)
def collect_data(env, policy, buffer, steps):
for _ in range(steps):
collect_step(env, policy, buffer)
initial_collect_steps = 100
collect_data(train_env, random_policy, replay_buffer, initial_collect_steps)
collect_step()은 주어진 환경에서 주어진 정책을 사용해서 데이터를 수집합니다.
collect_data() 함수를 사용해서 여러 회 (steps)의 데이터 수집 작업을 수행합니다.
3) 데이터셋 만들기¶
예제1¶
dataset = replay_buffer.as_dataset(
num_parallel_calls=3,
sample_batch_size=64,
num_steps=2).prefetch(3)
print(dataset)
<PrefetchDataset shapes: (Trajectory(step_type=(64, 2), observation=(64, 2, 4), action=(64, 2), policy_info=(), next_step_type=(64, 2), reward=(64, 2), discount=(64, 2)), BufferInfo(ids=(64, 2), probabilities=(64,))), types: (Trajectory(step_type=tf.int32, observation=tf.float32, action=tf.int64, policy_info=(), next_step_type=tf.int32, reward=tf.float32, discount=tf.float32), BufferInfo(ids=tf.int64, probabilities=tf.float32))>
TFUniformReplayBuffer 객체의 as_dataset() 메서드는 버퍼로부터 주어진 형식으로 만들어진 데이터셋을 반환하도록 합니다.
예제2¶
iterator = iter(dataset)
print(iterator.next())
(Trajectory(step_type=<tf.Tensor: shape=(64, 2), dtype=int32, numpy=
array([[1, 1],
[1, 1],
...
[1, 1],
[1, 1]], dtype=int32)>, observation=<tf.Tensor: shape=(64, 2, 4), dtype=float32, numpy=
array([[[ 1.28562853e-03, 1.83478072e-01, 1.37261108e-01,
3.56368721e-01],
[ 4.95519023e-03, -1.33012347e-02, 1.44388482e-01,
6.88989639e-01]],
...
[[ 6.29028752e-02, 7.78079510e-01, -3.83617505e-02,
-1.15275955e+00],
[ 7.84644634e-02, 9.73680317e-01, -6.14169426e-02,
-1.45722055e+00]]], dtype=float32)>, action=<tf.Tensor: shape=(64, 2), dtype=int64, numpy=
array([[0, 0],
[1, 1],
...
[0, 1],
[1, 0]])>, policy_info=(), next_step_type=<tf.Tensor: shape=(64, 2), dtype=int32, numpy=
array([[1, 1],
[1, 1],
...
[1, 1],
[1, 1]], dtype=int32)>, reward=<tf.Tensor: shape=(64, 2), dtype=float32, numpy=
array([[1., 1.],
[1., 1.],
...
[1., 1.],
[1., 1.]], dtype=float32)>, discount=<tf.Tensor: shape=(64, 2), dtype=float32, numpy=
array([[1., 1.],
[1., 1.],
...
[1., 1.],
[1., 1.]], dtype=float32)>), BufferInfo(ids=<tf.Tensor: shape=(64, 2), dtype=int64, numpy=
array([[69, 70],
[33, 34],
...
[55, 56],
[19, 20]])>, probabilities=<tf.Tensor: shape=(64,), dtype=float32, numpy=
array([0.01010101, 0.01010101, 0.01010101, 0.01010101, 0.01010101,
0.01010101, 0.01010101, 0.01010101, 0.01010101, 0.01010101,
0.01010101, 0.01010101, 0.01010101, 0.01010101, 0.01010101,
0.01010101, 0.01010101, 0.01010101, 0.01010101, 0.01010101,
0.01010101, 0.01010101, 0.01010101, 0.01010101, 0.01010101,
0.01010101, 0.01010101, 0.01010101, 0.01010101, 0.01010101,
0.01010101, 0.01010101, 0.01010101, 0.01010101, 0.01010101,
0.01010101, 0.01010101, 0.01010101, 0.01010101, 0.01010101,
0.01010101, 0.01010101, 0.01010101, 0.01010101, 0.01010101,
0.01010101, 0.01010101, 0.01010101, 0.01010101, 0.01010101,
0.01010101, 0.01010101, 0.01010101, 0.01010101, 0.01010101,
0.01010101, 0.01010101, 0.01010101, 0.01010101, 0.01010101,
0.01010101, 0.01010101, 0.01010101, 0.01010101], dtype=float32)>))
iter() 함수를 사용해서 데이터셋을 반복 가능한 객체로 변환하고,
next()를 사용해서 수집한 데이터를 확인할 수 있습니다.