Contents
- TF-Agents 모듈 임포트하기
- TF-Agents 환경 준비하기
- TF-Agents 환경 살펴보기
- TF-Agents 행동 반영하기
- TF-Agents 환경 Wrapper 사용하기
- TF-Agents 에이전트 구성하기
- TF-Agents 정책 다루기
- TF-Agents 정책 평가하기
- TF-Agents 데이터 수집하기
- TF-Agents 에이전트 훈련하기
Tutorials
- Python Tutorial
- NumPy Tutorial
- Matplotlib Tutorial
- PyQt5 Tutorial
- BeautifulSoup Tutorial
- xlrd/xlwt Tutorial
- Pillow Tutorial
- Googletrans Tutorial
- PyWin32 Tutorial
- PyAutoGUI Tutorial
- Pyperclip Tutorial
- TensorFlow Tutorial
- Tips and Examples
TF-Agents 정책 평가하기¶
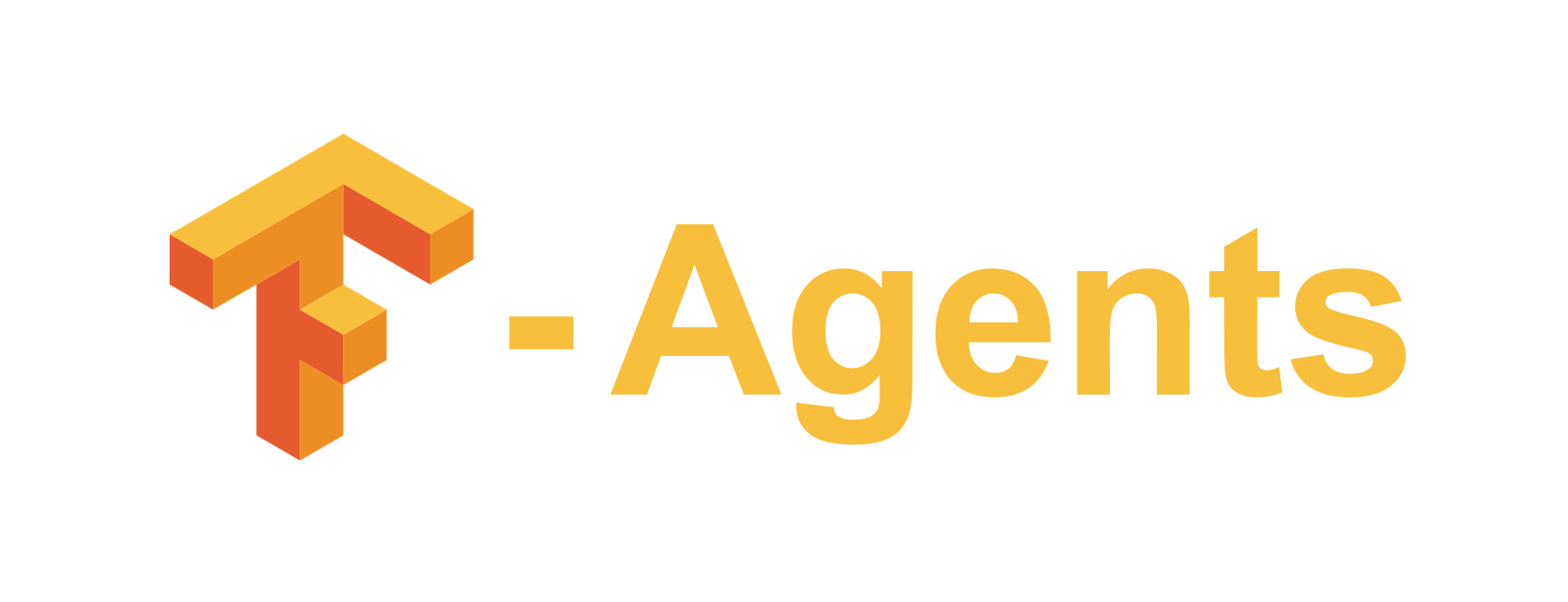
정책 (Policy)은 강화학습의 환경에서 에이전트가 동작하는 방식입니다.
이 페이지에서는 TF-Agents 정책 다루기에서 만들었던 에이전트의 정책을 평가하는 과정을 소개합니다.
■ Table of Contents
1) 정책 만들기¶
예제¶
import tensorflow as tf
from tf_agents.environments import suite_gym
from tf_agents.environments import tf_py_environment
from tf_agents.agents.dqn import dqn_agent
from tf_agents.networks import q_network
from tf_agents.utils import common
from tf_agents.policies import random_tf_policy
env_name = 'CartPole-v0'
train_py_env = suite_gym.load(env_name)
eval_py_env = suite_gym.load(env_name)
train_env = tf_py_environment.TFPyEnvironment(train_py_env)
eval_env = tf_py_environment.TFPyEnvironment(eval_py_env)
q_net = q_network.QNetwork(
train_env.observation_spec(),
train_env.action_spec(),
fc_layer_params=(100,))
optimizer = tf.compat.v1.train.AdamOptimizer(learning_rate=1e-3)
train_step_counter = tf.Variable(0)
agent = dqn_agent.DqnAgent(
train_env.time_step_spec(),
train_env.action_spec(),
q_network=q_net,
optimizer=optimizer,
td_errors_loss_fn=common.element_wise_squared_loss,
train_step_counter=train_step_counter)
agent.initialize()
eval_policy = agent.policy
collect_policy = agent.collect_policy
random_policy = random_tf_policy.RandomTFPolicy(train_env.time_step_spec(),
train_env.action_spec())
TF-Agents 정책 다루기에서 설명했던대로 eval_policy, collect_policy는 에이전트의 정책입니다.
또한 random_policy는 에이전트와 독립적이며, train_env의 특정 TimeStep에 대해 임의의 행동을 반환하는 정책입니다.
2) 정책 평가 함수 만들기¶
예제¶
def compute_avg_return(environment, policy, num_episodes=10):
total_return = 0.0
for _ in range(num_episodes):
time_step = environment.reset()
episode_return = 0.0
while not time_step.is_last():
action_step = policy.action(time_step)
time_step = environment.step(action_step.action)
episode_return += time_step.reward
total_return += episode_return
avg_return = total_return / num_episodes
return avg_return.numpy()[0]
compute_avg_return() 함수는 주어진 환경 (environment)과 정책 (policy) 인자에 대해 주어진 에피소드 (num_episodes) 동안의 평균 리턴 (보상의 총합의 평균)을 반환합니다.
total_return은 주어진 에피소드 동안 얻은 리턴의 총합이며, episode_return은 각 에피소드의 리턴 (보상의 총합)입니다.
TimeStep 인스턴스는 is_first(), is_mid(), is_last() 메서드를 포함합니다.
TimeStep 인스턴스의 is_last() 메서드는 해당 TimeStep이 에피소드의 마지막 TimeStep인지 여부를 반환합니다.
3) 정책의 평균 리턴 확인하기¶
예제¶
avg_returns = []
for _ in range(5):
avg_returns.append(compute_avg_return(eval_env, random_policy, 10))
print(avg_returns)
[25.7, 22.1, 22.9, 29.0, 20.4]
avg_returns 리스트의 각 값은 10회 에피소드 동안의 평균 리턴 값을 의미합니다.
eval_env 환경에서 random_policy는 평균 리턴값이 30보다 작음을 알 수 있습니다.
CartPole-v0 게임의 목표는 100회의 연속적인 시도에서 평균 195회의 평균 리턴을 획득하는 것입니다.
즉, 100회 이상의 연속된 에피소드에서 195회의 TimeStep 동안 막대기가 넘어지지 않도록 유지하는 것입니다.